CHURN MODEL
- Savvy Analytic Solutions | Atlanta
- Apr 13, 2021
- 1 min read
Updated: Aug 22, 2021
Data for this simulation was obtained from IBM.
OBJECTIVE
Use Customer Churn Prediction To help Customer Relationship Management (CRM)
Incentivize potential churners
Seize opportunity with non-churners
THE SURVEY DATA
BENEFITS
The predictions from the ML model can help in understanding the customers who might leave and their service. With this information the company can do the following:
Lower customer churn
Reduction of dispute calls
Reduce the operational cost of call centers
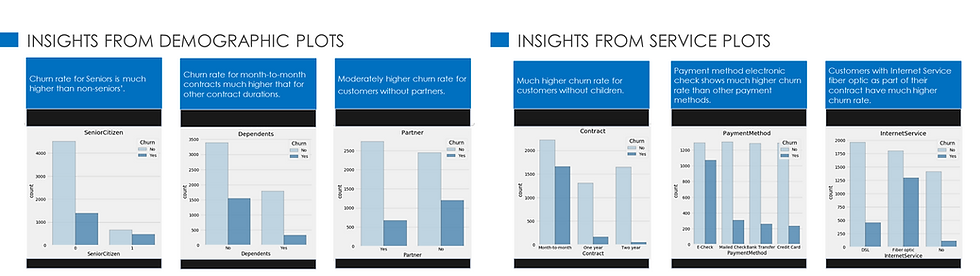
churn = -1.41 + 0.14(senior_citizen-yes) -0.03(tenure) + 0.92(internet_service-dsl) + 1.82(internet-service-fiber-optic) -0.88(contract-one-year) -1.68(contract-two-year)
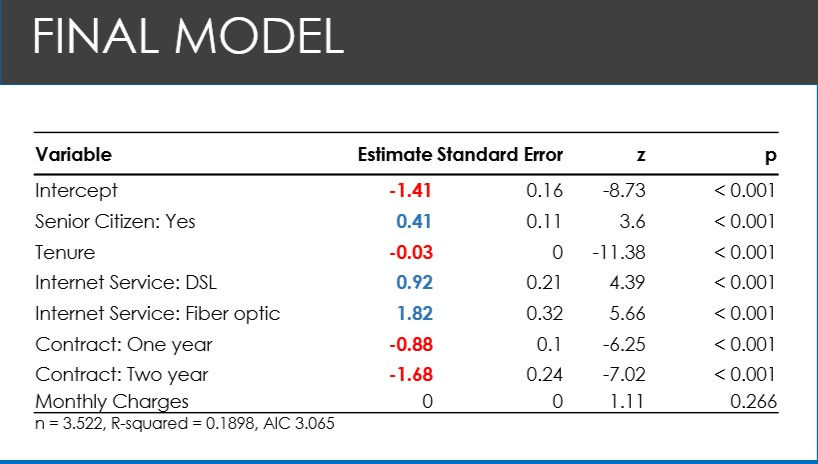
CONCLUSIONS: FACTORS OF IMPORTANCE
Tenure
Contract
Paperless Billing
Monthly Charges
Internet Service
CONCLUSIONS
Customers with month-to-month contracts are less likely to churn.
Customers with internet service, in particular fiber optic service, are more likely to churn.
Customers who have been with the company longer or have paid more in total are less likely to churn.
IMPLEMENTATION
The predictions from the ML model can help in understanding the customers who might leave and their service. With this information the company can do the following:
Daily Feed: Relationship Managers(RM) get a daily feed on who has the propensity to churn and what are the influencing factors
Customer Engagement: Triggers a conversation with the customer and understand their pain points and possibly rectify the situation
Lifetime Value (LTV): Increases customer entanglement as the RM would reach out to them anticipating issues will eventually increase their lifetime value
Comments